Únase a getAbstract para acceder al resumen.
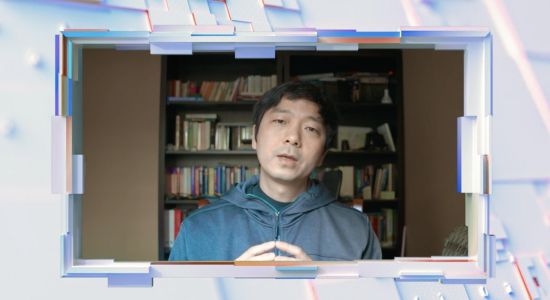
Únase a getAbstract para acceder al resumen.
Siddhartha Chaturvedi and Hoifung Poon
Biomedical Natural Language Processing
Microsoft, 2021
¿De qué se trata?
Biomedical Natural Language Processing (NLP) is transforming medicine.
Recommendation
Biomedical Natural Language Processing (NLP) stands on the cutting edge of artificial intelligence (AI), poised to transform medicine. New technologies can extract patient data in granular detail and at large scales, which may lead to custom, patient-specific treatments. In this Microsoft Tech Minutes video, Microsoft’s Siddhartha Chaturvedi talks with Dr. Hoifung Poon, senior director of Biomedical NLP at Microsoft Health Futures about NLP’s current and future abilities.
Take-Aways
- Biomedical Natural Language Processing (NLP) stands on the cutting edge of artificial intelligence.
- NLP will transform medicine.
- NLP still faces technical challenges.
Summary
Biomedical Natural Language Processing (NLP) is on the cutting edge of artificial intelligence.
Investment and research in natural language processing bias toward general domains – such as the news media – rather than specific domains like medicine, in which large amounts of information are available, but have proven difficult to analyze on the scale required. The development of Biomedical NLP aims to deal with biomedicine’s massive amounts of information.
“The disruption from new technology has made it possible to attain high definition patient data at scale. For example, the cost of sequencing a human genome has just dropped below $1,000, making it broadly applicable to cancer and other disease.” (Dr. Hoifung Poon)
Furthering NLP, most patient data is now available in digital form, and Electronic Medical Records have become the norm. Biomedical NLP can provide a detailed, accurate picture of patient data, and help usher in an era of precision medicine featuring custom treatments developed for individual patients.
NLP will transform medicine.
NLP’s capacity to access, process and analyze patient data means medicine will become personalized and incredibly precise. And yet, even more information exists that can be accessed. For example, every day sees the publication of some 4,000 papers in biomedicine – around a million per year. Machine reading can access the information contained therein, and quickly.
Machine reading could, for example, extract knowledge about the coronavirus to determine the relationship between the disease and its symptoms, the virus itself and the human immune response. Scientists can use that data analysis to develop new treatments. In addition, technicians can exploit regulatory changes to apply machine reading to Electronic Medical Records and clinical devices to generate longitudinal patient profiles. This step will prove crucial for advancing clinical research. This process is the heart of the “real-world evidence” research model that profoundly affects patient care.
NLP still faces technical challenges.
Biomedical research engages far more complex linguistic issues than general domain data, such as the news media. General domain NLP models often overlook or misinterpret biomedical vocabulary, such as disease names. To avoid that problem, Biomedical NLP training utilized a medical dataset with millions of abstracts and over three billion words. NLP demanded this training because – unlike most natural language processing models – NLP needs to extract complicated relationships between disparate paragraphs.
“Biomedical NLP also faces additional machine learning challenges. Standard Supervised Learning requires labeled examples and in biomedicine, labeling such examples requires deep domain expertise.” (Dr. Hoifung Poon)
The need for specialized expertise raises difficulties around crowdsourcing learning samples – as general domain models do. Relying on advanced models, Biomedical NLP uses Self-Supervised Learning with unlabeled samples within a larger context called “Deep Probabilistic Logic.” By doing so, Biomedical NLP can analyze “noisy” and potentially contradictory data and combine them in a graphic representation. It can also develop new ways of self-supervising as it proceeds.
NLP constitutes a “human-computer symbiosis.” Biomedical machine reading, for example, can sort through thousands of published articles and find relevant information, which the person overseeing or “curating” the process can select and verify. And – exemplifying the breakthroughs NLP promises – the curator can now perform this function in a reasonable and actionable time frame.
About the Speakers
Siddhartha Chaturvedi collaborated in building mosquito-catching robots, T cell–based diagnostics and domain-specific natural language processing. Dr. Hoifung Poon is senior director of Biomedical NLP at Microsoft Health Futures.
This document is restricted to personal use only.
Comment on this summary